ViCo
Conversational Head Generation Challenge
held in conjunction with ACM Multimedia 2023
In face-to-face communication, the speaker transmits the verbal and non-verbal messages explicitly by keeping pace with the listener's reactions, and the listener receives the message and provides real-time responsive feedback to the speaker through non-verbal behavior (e.g., nod, smile, shake, etc.). Generating vivid talking head video and proper responsive listening behavior are both essential for digital humans during face-to-face human-computer interaction.
This challenge is based on our extended `ViCo'' dataset, which is so far the first video conversation dataset containing face-to-face dialogue video clips in various scenarios. In constract to the challenge in 2022, more videos have collected to enable the use of more advance machine learning methods. We aim to bring the face-to-face interactive head video generation into a visual competition in this challenge. A comprehensive collection of conversational video clips are selected from YouTube, containing two people's frontal face by strictly following the principle that a video clip contains only uniquely identified listener and speaker, and requires the listener has responsive non-verbal feedback to speaker on the content of the conversation.
ViCo conversational head generation challenge is organized in conjunction with ACM Multimedia 2023. The challenge includes two tracks:
- Vivid Talking Head Video Generation conditioned on the identity and audio signals of the speaker.
- Responsive Listening Head Video Generation conditioned on the identity of the listener and with responding to the speaker's behaviors in real-time.
Individuals and teams with top submissions will present their work at the workshop. We also encourage every team to upload a paper (may consist of up to 4 pages) that briefly describes their system. The paper format follows ACM proceeding style.
If you would like to receive notifications about the challenge (e.g. challenge process, launch time, deadline, awards announcement), please subscribe through this url.
If there are any questions, please let us know by raising an issue or contact us <vico-challenge[at]outlook[dot]com>.
All of the videos in our ViCo dataset are collected from the online video sharing platform YouTube. Rich video clips are contained in the dataset. These clips are collected from various face-to-face conversational scenarios, where bi-directional information flow is highlighted between two people. All video clips are manually checked/labeled by the production expert team.
Show example videos from our ViCo dataset.
More details about ViCo dataset, please refer to:Mohan Zhou, Yalong Bai, Wei Zhang, Ting Yao, Tiejun Zhao, Tao Mei. "Responsive Listening Head Generation: A Benchmark Dataset and Baseline".
[ECCV 2022] [Project]
Train+Test Set:
Train Set: OneDrive
Validation Set (same to 2022): OneDrive
Test Set: OneDrive
**Note: Except for the driver part, all other parts (e.g. render) can use additional data, but teams need to declare the pretrained model or additional data used when submission. For example, the use of a Talking Head Generation data is not allowed, but the use of pretrained data / model to adjust the render is allowed.
Guidelines
In Train Set, for each track, the data consists of three parts:
videos/*.mp4
: all videos without audio trackaudios/*.wav
: all audios-
*.csv
: return meta data about all videos/audiosName Type Description video_id str ID of video uuid str ID of video sub-clips speaker_id int ID of speaker listener_id int ID of listener, only in listening_head audios/{uuid}.wav
can be identified, and the listener's video isvideos/{uuid}.listener.mp4
, the speaker's video isvideos/{uuid}.speaker.mp4
.
output/
directory.The inputs consist of these parts:
videos/*.mp4
: speaker videos, only in listening_headaudios/*.wav
: all audiosfirst_frames/*.jpg
: first frames of expected listener/speaker videosref_images/(\d+).jpg
: reference images by person id*.csv
: return meta data about all videos/audios, same to CSVs in Train Set
Example generations on train set:
Please refer to our Leaderboard Page.
The quality of generated videos will be quantitative evaluated from the prespectives of visual quality, naturalness, and task-specified goals:
Category | Metric | Note |
---|---|---|
Visual Quality | CPBD | image sharpness |
FID | distance between synthetic and real data distributions | |
SSIM | perceptual similarity of image contrast, luminance and structure | |
PSNR | the error of synthetic data compared to real | |
CSIM | identity preservation from ArcFace | |
Naturalness | ExpL1 | $L_1$ distance of expression coefficients from 3DMM reconstruction |
PoseL1 | $L_1$ distance of translation/rotation coefficients from 3DMM reconstruction | |
Speaker-specified | AVOffset | the synchronization offset of lip motion with input audio |
AVConf | the confidence of AVOffset | |
LipLMD | the lip landmark distance | |
Listener-specified | ExpFD | Fréchet distance in expression coefficients from 3DMM reconstruction |
PoseFD | Fréchet distance in translation/rotation coefficients from 3DMM reconstruction |
The submission deadline is at (23:59) 11:59 p.m. of the stated deadline date (Anywhere on Earth).
Dataset available for download (training set) | March 27, 2023 |
Test set of each track available for download | June 5, 2023 |
Challenge submissions deadline | |
Evaluation results and challenge award announce | |
Paper submission deadline** | July 14, 2023 |
MM Grand Challenge Camera Ready papers due** | July 31, 2023 |
**: hard deadline, will not be tolerated
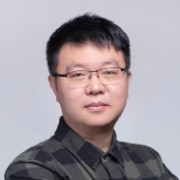
Yalong Bai
JD Explore Academy
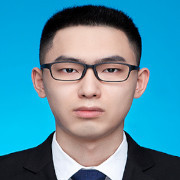
Mohan Zhou
HIT, Harbin, China
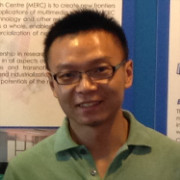
Wei Zhang
JD Explore Academy
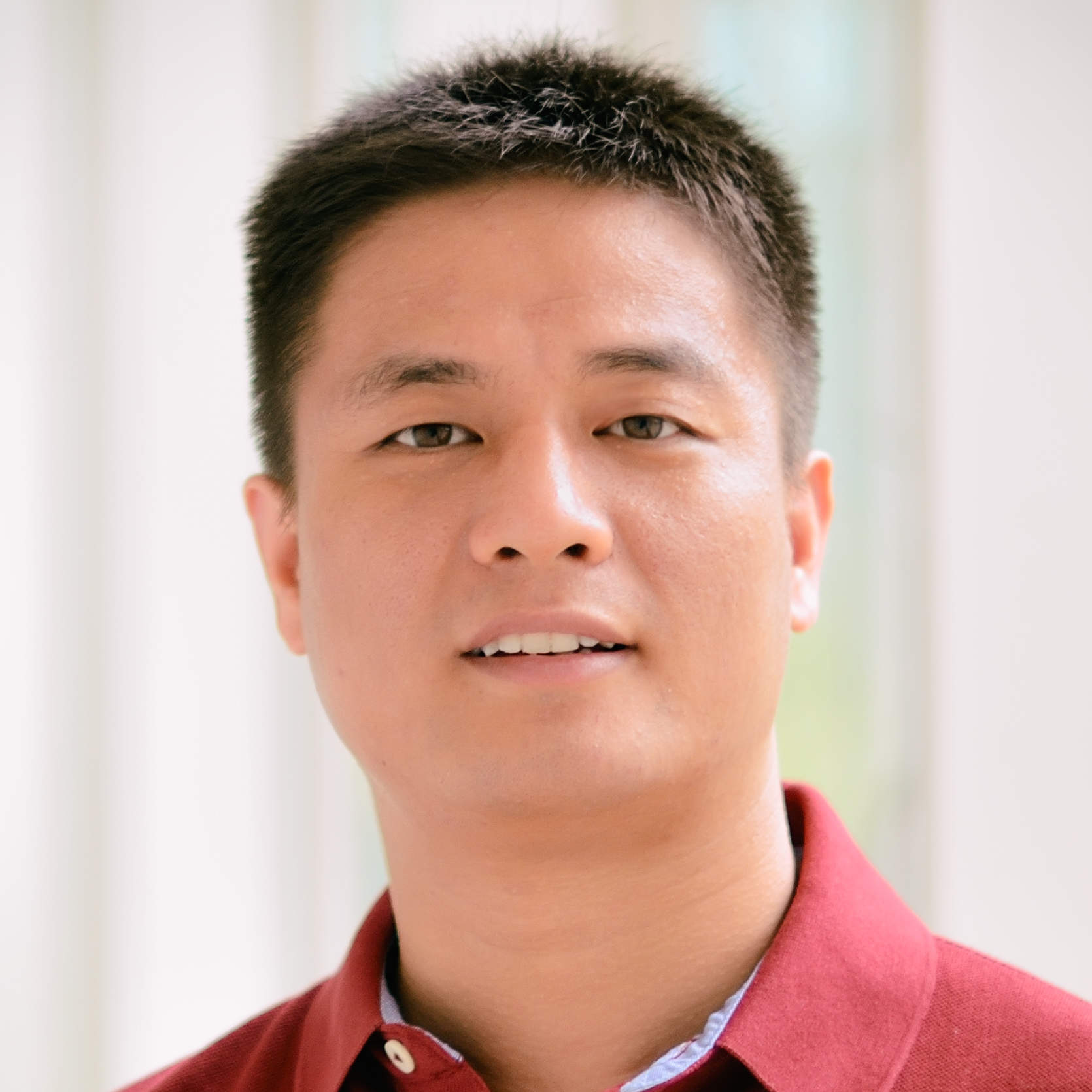
Ting Yao
JD Explore Academy
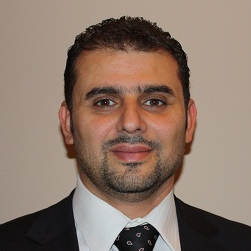
Abdulmotaleb El Saddik
University of Ottawa
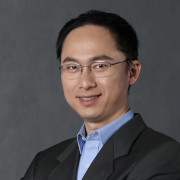
Xiaodong He
JD Explore Academy
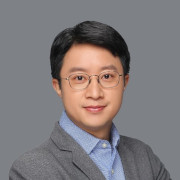
Tao Mei
JD Explore Academy